Note
Go to the end to download the full example code
05. Image deblurring#
Deblurring is the process of removing blurring effects from images, caused for example by defocus aberration or motion blur.
In forward mode, such blurring effect is typically modelled as a 2-dimensional convolution between the so-called point spread function and a target sharp input image, where the sharp input image (which has to be recovered) is unknown and the point-spread function can be either known or unknown.
In this tutorial, an example of 2d blurring and deblurring will be shown using
the pylops.signalprocessing.Convolve2D
operator assuming knowledge
of the point-spread function.
import matplotlib.pyplot as plt
import numpy as np
import pylops
Let’s start by importing a 2d image and defining the blurring operator
im = np.load("../testdata/python.npy")[::5, ::5, 0]
Nz, Nx = im.shape
# Blurring guassian operator
nh = [15, 25]
hz = np.exp(-0.1 * np.linspace(-(nh[0] // 2), nh[0] // 2, nh[0]) ** 2)
hx = np.exp(-0.03 * np.linspace(-(nh[1] // 2), nh[1] // 2, nh[1]) ** 2)
hz /= np.trapz(hz) # normalize the integral to 1
hx /= np.trapz(hx) # normalize the integral to 1
h = hz[:, np.newaxis] * hx[np.newaxis, :]
fig, ax = plt.subplots(1, 1, figsize=(5, 3))
him = ax.imshow(h)
ax.set_title("Blurring operator")
fig.colorbar(him, ax=ax)
ax.axis("tight")
Cop = pylops.signalprocessing.Convolve2D(
(Nz, Nx), h=h, offset=(nh[0] // 2, nh[1] // 2), dtype="float32"
)
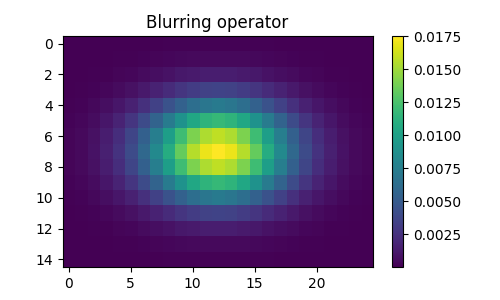
We first apply the blurring operator to the sharp image. We then try to recover the sharp input image by inverting the convolution operator from the blurred image. Note that when we perform inversion without any regularization, the deblurred image will show some ringing due to the instabilities of the inverse process. Using a L1 solver with a DWT preconditioner or TV regularization allows to recover sharper contrasts.
imblur = Cop * im
imdeblur = pylops.optimization.leastsquares.normal_equations_inversion(
Cop, imblur.ravel(), None, maxiter=50 # solvers need 1D arrays
)[0]
imdeblur = imdeblur.reshape(Cop.dims)
Wop = pylops.signalprocessing.DWT2D((Nz, Nx), wavelet="haar", level=3)
Dop = [
pylops.FirstDerivative((Nz, Nx), axis=0, edge=False),
pylops.FirstDerivative((Nz, Nx), axis=1, edge=False),
]
DWop = Dop + [Wop]
imdeblurfista = pylops.optimization.sparsity.fista(
Cop * Wop.H, imblur.ravel(), eps=1e-1, niter=100
)[0]
imdeblurfista = imdeblurfista.reshape((Cop * Wop.H).dims)
imdeblurfista = Wop.H * imdeblurfista
imdeblurtv = pylops.optimization.sparsity.splitbregman(
Cop,
imblur.ravel(),
Dop,
niter_outer=10,
niter_inner=5,
mu=1.5,
epsRL1s=[2e0, 2e0],
tol=1e-4,
tau=1.0,
show=False,
**dict(iter_lim=5, damp=1e-4)
)[0]
imdeblurtv = imdeblurtv.reshape(Cop.dims)
imdeblurtv1 = pylops.optimization.sparsity.splitbregman(
Cop,
imblur.ravel(),
DWop,
niter_outer=10,
niter_inner=5,
mu=1.5,
epsRL1s=[1e0, 1e0, 1e0],
tol=1e-4,
tau=1.0,
show=False,
**dict(iter_lim=5, damp=1e-4)
)[0]
imdeblurtv1 = imdeblurtv1.reshape(Cop.dims)
Finally we visualize the original, blurred, and recovered images.
# sphinx_gallery_thumbnail_number = 2
fig = plt.figure(figsize=(12, 6))
fig.suptitle("Deblurring", fontsize=14, fontweight="bold", y=0.95)
ax1 = plt.subplot2grid((2, 5), (0, 0))
ax2 = plt.subplot2grid((2, 5), (0, 1))
ax3 = plt.subplot2grid((2, 5), (0, 2))
ax4 = plt.subplot2grid((2, 5), (1, 0))
ax5 = plt.subplot2grid((2, 5), (1, 1))
ax6 = plt.subplot2grid((2, 5), (1, 2))
ax7 = plt.subplot2grid((2, 5), (0, 3), colspan=2)
ax8 = plt.subplot2grid((2, 5), (1, 3), colspan=2)
ax1.imshow(im, cmap="viridis", vmin=0, vmax=250)
ax1.axis("tight")
ax1.set_title("Original")
ax2.imshow(imblur, cmap="viridis", vmin=0, vmax=250)
ax2.axis("tight")
ax2.set_title("Blurred")
ax3.imshow(imdeblur, cmap="viridis", vmin=0, vmax=250)
ax3.axis("tight")
ax3.set_title("Deblurred")
ax4.imshow(imdeblurfista, cmap="viridis", vmin=0, vmax=250)
ax4.axis("tight")
ax4.set_title("FISTA deblurred")
ax5.imshow(imdeblurtv, cmap="viridis", vmin=0, vmax=250)
ax5.axis("tight")
ax5.set_title("TV deblurred")
ax6.imshow(imdeblurtv1, cmap="viridis", vmin=0, vmax=250)
ax6.axis("tight")
ax6.set_title("TV+Haar deblurred")
ax7.plot(im[Nz // 2], "k")
ax7.plot(imblur[Nz // 2], "--r")
ax7.plot(imdeblur[Nz // 2], "--b")
ax7.plot(imdeblurfista[Nz // 2], "--g")
ax7.plot(imdeblurtv[Nz // 2], "--m")
ax7.plot(imdeblurtv1[Nz // 2], "--y")
ax7.axis("tight")
ax7.set_title("Horizontal section")
ax8.plot(im[:, Nx // 2], "k", label="Original")
ax8.plot(imblur[:, Nx // 2], "--r", label="Blurred")
ax8.plot(imdeblur[:, Nx // 2], "--b", label="Deblurred")
ax8.plot(imdeblurfista[:, Nx // 2], "--g", label="FISTA deblurred")
ax8.plot(imdeblurtv[:, Nx // 2], "--m", label="TV deblurred")
ax8.plot(imdeblurtv1[:, Nx // 2], "--y", label="TV+Haar deblurred")
ax8.axis("tight")
ax8.set_title("Vertical section")
ax8.legend(loc=5, fontsize="small")
plt.tight_layout()
plt.subplots_adjust(top=0.8)
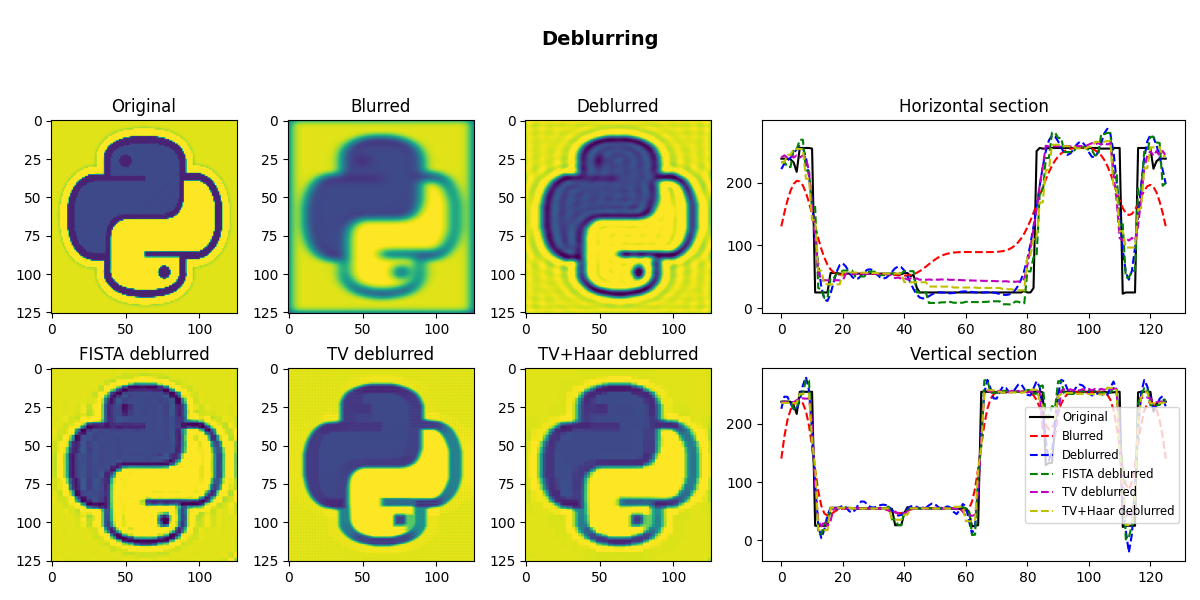
Total running time of the script: (0 minutes 6.778 seconds)